les Nouvelles April 2024 Article of the Month:
Will Artificial Intelligence Shape The Future Of Technology Transfer? A Guide For Licensing Professionals
Technology Transfer and Patent Specialist
Cancer Therapy Evaluation Program
Division of Cancer Treatment and Diagnosis
National Cancer Institute
Rockville, MD
Office of Technology Transfer
National Institutes of Health
Bethesda, MD
Abstract
Unleashed Artificial Intelligence (AI) is a multidisciplinary technology that is spreading in our daily life from email filtration for spam and biomedical field applications to legal services. The technology transfer field requires well-organized and promptly managed agreement (contract) management and invention commercialization to be truly effective. AI has been considered one of the alternative tools for the complex contract management system. In this review article, we examine the current role of AI in technology transfer and review the capabilities to understand its potential future impact in this field better.
1. Introduction
Artificial intelligence is an intellectual, technological tool that can perform tasks that require cognitive human brain functions, including knowledge interpretation, judgment, reasoning, and decision-making in an automated way. AI has an ancient history. Aristotle, St. Thomas Aquinas, William of Ockham, René Descartes, Thomas Hobbes, and Gottfried W. Leibniz questioned the basic cognitive operations and their automation.1, 2
Artificial intelligence (AI) is a multidisciplinary technology, including statistics, mathematics, economics, biology/neuroscience, psychology/ cognitive science, logic, philosophy, evolution, and computer science.4 Interestingly, it is a trainable technology that can interpret and learn from giant data sets how to perform specific tasks and create solutions by mimicking human mind adaptation. Al technology has been growing rapidly, and there has been tremendous investment in this field. The total investment in AI technology was $168.39 billion in 2022 (Figure 1). According to Pitchbook’s most recent records, there are currently 38,969 companies working in the AI technology field, and 16,827 of the AI companies that is, equal to 45 percent, are in the United States. The federal government has been supporting AI-based technology development and adoption in the United States with executive orders and guidance. For example, on November 17, 2020, an executive order for the “Guidance for Regulation of Artificial Intelligence Applications” was released by the U.S. government. This executive order aims to guide all federal agencies in informing the development of regulatory and nonregulatory approaches regarding technologies and industrial sectors that are empowered or enabled by AI and considers ways to reduce barriers to developing and adopting AI technologies. On January 1, 2021, the National AI Initiative Act 2020 (Division E, Sec. 5001) also became law to provide a coordinated program across the federal government to accelerate AI research and application.
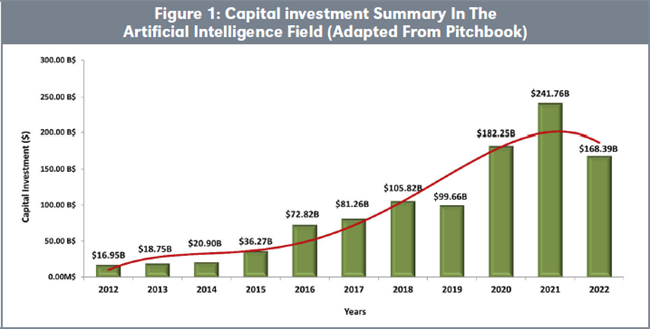
We searched companies that are involved in AI and Machine Learning (ML) within the contract management field in Pitchbook to understand the current landscape of the AI position in agreement management. According to Pitchbook’s recent data, there are only 55 companies out of 38,969 that work in the contract management field. As seen in Figure 2, 45 percent of AI-based contract management companies are from the United States, and Europe has 33 percent.
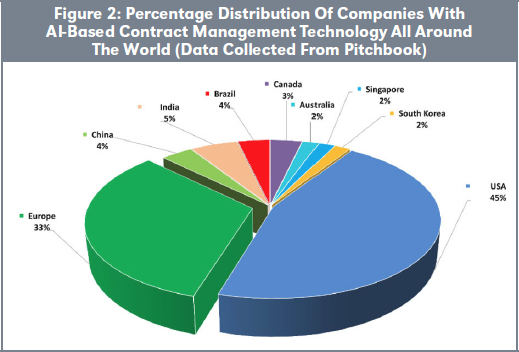
This study aims to provide a general overview of AI currently in use in contract management and to draw a broad picture of the potential role of AI in the future of technology transfer offices.
2. Methods
We used multiple databases and the resources listed below to understand the current and potential future role of AI in the technology transfer field.
2.1. Databases Used In This Study
To explore companies that work with AI and ML in the contract management field and explore published articles and opinions, we used multiple databases as listed below.
- Pitchbook (https://pitchbook.com): This platform provides access to private and public capital market data for 3.3 million companies, and it delivers data regarding company investors, deals, mergers and acquisitions, funds, advisors, and people. Through its daily newsletter and quarterly reports, the company also provides commentary and analysis of current events, trends, and issues relevant to the capital markets.
Pitchbook was queried to identify companies that work with AI and ML in the contract management field. We sorted companies according to their foundation year, plotted the companies' number of years, and examined the polynomial trendline of the plots to have a better understanding of the trend in AI-based technologies in contract management. We have also examined the investment in the AI-based contract management field.
- LinkedIn: LinkedIn is the largest professional network on the internet. LinkedIn was used to collect information and opinions from technology transfer experts about the current and future applications of AI and ML in the contract management field. There are great discussion forums that include an expert’s current experience in AI’s application in contract management and her forecast about AI’s potential in the future of the technology transfer arena. LinkedIn also has published blog articles about the current usage of AI-based technologies in the technology transfer field by the topic experts.
- Association of University Technology Managers (AUTM): AUTM is an association of over 3,100 technology transfer professionals. It is a platform to create connections between academic and industry technology transfer professionals from 60 countries. AUTM initiated an internal forum about AI-based technologies in technology transfer offices in universities and law firms. We used information from this AUTM forum about the current and future role of AI in contract management.
- Webinar Series about AI-based Technologies:
We participated in the webinar series listed below to collect information about the general application of AI-based technologies, its advantages, and the parts that need to be further improved.
- NCI Webinar Series: NCI organized a webinar series titled “The United States and European Union Artificial Intelligence Engagement Series,” which was held on July 25, July 26, and July 28, 2023. This webinar series consisted of three topics:
- United States and European Union Artificial Intelligence Engagement Series: Privacy Preserving AI, July 25, 2023
- United States and European Union Artificial Intelligence Engagement Series: Community/ Patient Engaged AI for Biomedical Research, July 26, 2023
- United States and European Union AI Engagement Series: Ethical AI and the Inclusion of Underserved Communities, July 28, 2023
- AUTM Webinar: Generative AI has Arrived: Essential Knowledge for TTOs, May 11, 2023
- Google Scholar: Google Scholar allows access to indexes of the full text or metadata of scholarly literature across an array of publishing formats and disciplines. An AI-related literature search was made using Google Scholar.
3. History of AI and its Current Applications
AI has an ancient history. In Socrates’ time (~400 BC), the availability of an algorithm was questioned. In Aristotle’s time (300 BC), various types of reasoning approaches were developed to reach conclusions using initial premises, which created the deductive reasoning approach as a standard inference technique in predicate and propositional logic.1 In Rene Descartes’s era (1596-1650), mind-body dualism came to light, and free will was introduced as a piece of the mind. Today, all AI discussions conclude that AI will never have human intelligence’s free will; therefore, there will always be goal assignments by humans for the AI systems. On the other hand, Gottfried Wilhelm Leibnitz, a German philosopher and mathematician (1646–1716), supported the materialist nature of the mind and hypothesized that ordinary physical processes operate the mind. This means that machines can perform mind processes according to today’s concept.
The development history of AI has three waves, as seen in Figure 3. These waves were identified based on the AI field’s interest, funding, research, and development activities. John McCarthy, the first scientist to define “artificial intelligence,” and his colleagues founded the field of AI in 1956 at a Dartmouth College conference. This conference is called a starting point of AI. Therefore, it is called the First Summer of AI.1 During the first summer of AI, there had been a significant rise in the technology’s development and use. However, the same interest brought vulnerabilities to the technology due to high expectations and frustrations. Therefore, in the early 1970s, funding and interest in AI dropped, and AI’s first winter started. For similar reasons, there have been three waves in the AI field, and we are currently in the third wave and third summer of AI.2-4
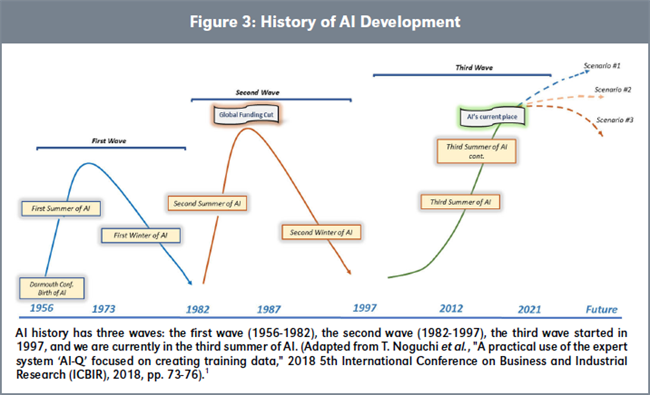
AI has many applications, and its involvement in our daily activities increases every day. One great example is that AI technology can manage our whole trip, from flight ticket purchases to the arrival at the destination. Al can book a flight ticket, decide how much the flight will cost, monitor the airport, and assist the pilot until a passenger reaches the destination. More interestingly, AI technology determines the fate of an individual by deciding if a person is eligible for a loan, welfare, or a particular job. AI technology is currently in use for self-driving cars. AI oversees and manages an individual’s social media activities by recommending what to see on social media in online shops and on YouTube. AI technology does not just make a simple recommendation but creates a tailor-made social media environment to increase consumption. AI is not a dream anymore, but a new reality in our daily life.3
4. Methods to Develop AI-based Technologies
There are two approaches to developing AI technology. One of the approaches uses symbolism, i.e., transforms knowledge into symbols and builds an algorithm based on the symbols. It is called Machine Learning, which allows Al to learn by using algorithms to identify the patterns from the data. AI and ML are intermingled terms. In comparison, AI refers to a trained, intelligent system. ML refers to training methods that use algorithms to automatically learn insights and recognize patterns from data, applying that learning to make increasingly better decisions.
The second approach focuses on neural networks called Deep Learning, an arm of ML, that uses human knowledge, transfers knowledge into symbols, and stimulates learning ability in a computer. Deep Learning gives AI the ability to mimic the human brain’s neural network. In this way, AI senses patterns in data and can train itself. This is also called Unsupervised Learning and one example of this is Google News which groups new stories on the web and puts them into collective news stories.4, 5 Machine Learning and Deep Learning have different ways for the acquisition of knowledge. The first one focuses on the role of logic, while the second one focuses on experience and learning.
In 1969, the neural network Deep Learning was considered incapable of processing complex equations. Therefore, it was abandoned, and symbolism became the dominant approach. The United States took the lead in AI development, and most AI technology uses symbolism in the U.S. However, Asian countries, including Japan and Korea, prefer to develop AI technology using the neural network approach, since AI technology development with symbolism has difficulty converting data in Asian languages into symbols.3
There are four types of AI with different use areas: Reactive AI, Limited Memory AI, Theory of Mind AI, and Self-aware AI.
The basic AI type is Reactive AI. Reactive AI is developed to use input for a predictable output, and machines with Reactive AI always perform the same way in the same situations. Reactive AI examples include chess players, spam filters, and the Netflix recommendation engine. Reactive AI has limited capabilities, and it cannot learn from experts.
The second group of AI is Limited Memory AI. A Limited Memory AI can learn from the past and build knowledge by observing or complying with data. This AI can use historical, observational data combined with pre-programmed information, make predictions, and perform complex tasks. Limited Memory AI is the most used AI. Autonomous vehicles use Limited Memory AI. As it is included in its name, Limited Memory AI has limited capacity.
The third group of AI is Theory of Mind AI, and it is not fully developed yet. However, this type of AI will understand humans and remember human emotions as they interact with humans and will be able to decide like humans. Dr. Cynthia Breazeal from MIT developed a robot head that could recognize emotional signals on human faces and mimic those emotions on its own face. Hanson Robotics from Hong Kong also developed a humanized robot that can recognize faces and respond to interactions with her facial expressions.
The fourth generation of AI is Self-Aware AI. It will be the most advanced type of AI with self-awareness and emotions. However, Self-aware AI has yet to be developed.6
5. AI’s Current Role in Technology Transfer
The technology transfer process starts with an invention. When there is an invention, the first step should be the invention disclosure by the inventor. Once the technology transfer office receives an invention disclosure form, the following steps are implemented: Evaluation of the invention, Protection, Marketing, Licensing, and Financial Return. This part of the article will present AI’s current use through the technology transfer processes mentioned above.
5.1. The Use of AI in Invention Evaluation
5.1.1. Patentability Evaluation: AI-based prior art search tools are excellent examples of AI in the technology transfer field. The patent prior art searching process is interactive and human-centered, requiring multiple searches and well-planned search management. A prior art search is for a determination as to whether a similar idea has been published in a filed patent. To answer this question, AI-based sophisticated tools can search giant databases in minutes, even though many are in foreign languages or non-standardized formats. Therefore, prior art search with AI-based tools is faster and more relevant due to its capacity to search more extensive databases.7 AI tools primarily rely on the data accumulated from thousands of human-based manual search results for prior art searches. During the webinar series titled “The United States and European Union Artificial Intelligence Engagement Series” organized by NCI, it is indicated that larger and better data quality means more accurate outcomes with AI-based tools.8 Therefore AI-mediated tools might generate accurate prior art search results if there is a large amount of high-quality data.
Another important element in the prior art search is taxonomies and search statements. If an AI system uses incorrect taxonomies to search prior art and the search statement is not well-defined, AI can create irrelevant results and present them as valid. Therefore, checking AI outcomes with manual search results is still vital. Even though AI-based tools can search multiple databases in minutes, it might still lead to biased results over others, and these biased results events might not be recognizable. Since all of the databases that AI searched may not be in the same format, there might be some blind spots when AI evaluates them. For this reason, there should always be validation by human experts with manual searches.
AI-based prior art search tools can reduce the time and cost of prior art searches, and patent examiners/ experts can save time by using AI-based tools.14 However, AI should be sufficient in these two crucial elements: (1) selecting relevant search queries and (2) creating the correct search statement. These two elements are required to understand the subject and the novelty of the application clearly. However, AI does not have the capability to perform these elements. Therefore, an AI-assisted search would require an expert to formulate a search statement because there are currently no practical AI algorithms to generate an efficient search statement.14
5.1.2. Marketing Evaluation and Marketing of Invention: AI is a rising star in marketing and plays a crucial role in business development since there is excessive data, advanced-level machine learning algorithms, models to predict consumer trends, and, more importantly, lower costs. There is a tremendous amount of AI use in marketing. Amazon’s Prime delivery, Domino’s Pizza’s experiment in autonomous cars and delivery robots, and Macy’s call center with natural language processing are AI-based marketing examples. AI can be used in three major steps of marketing: (1) marketing research, (2) marketing strategy, and (3) marketing action. Marketing research is an essential part of an invention evaluation, and AI has the power to accomplish this task.9
AI can have three levels of intelligence according to the requirement of the marketing task: (1) Mechanical AI can automatically connect data about the market, the environment, the firm, the competitors, and the customers; (2) Thinking AI can recognize patterns in data; and (3) Feeling AI can interact with humans, involving human input or analyzing human feelings and emotions. AI can identify competitors and predict market trends.
AI is a magical tool that can process high-quality market research data to complete various tasks, including identifying the target customer groups. AI can do all these essential marketing processes faster and more efficiently than humans.9, 10 Therefore, AI can be used as an efficient tool in the marketing analysis of an invention. AI can find target companies for marketing campaigns based on keywords, find competitors, and predict future market trends for an invention. However, like all other processes that run by AI, AI-based marketing tools also require excessive amounts of data for accurate marketing strategies,9 and outcomes still need to be cross-checked against other databases to ensure the accuracy of AI.11 It is always kept in mind that, despite the advantages of AI, lack of governance, data quality, and efficient employee training might cause risky business decisions and faulty marketing decisions.10
5.2. AI in Licensing and Agreement (Contract) Management: Federal legislation allows federal agencies to use various agreements and tools to create cooperation between federal and non-federal entities, including industrial for-profit organizations. For example, the average timeline for developing an oncology drug is approximately ten years.4 Collaboration becomes more crucial to overcome this extended time to create solutions for the public’s unmet medical needs, and it requires well-established agreements, in other terms, contracts. The National Cancer Institute is an excellent example of the government’s supportive role in collaboration with early-stage and big pharmaceutical companies to accelerate drug development.12 Technology transfer agreements, also called contracts, are listed, including but not limited to Cooperative Research and Development Agreements (CRADA), Material Transfer Agreements (MTA), Confidential Disclosure Agreements (CDA), Licensing Agreements, and Data Usage Agreements (DUA). All these agreements create a robust and very efficient information flow between universities, government organizations, nonprofit organizations, and profitable small or big companies. While Confidential Disclosure agreements (CDAs) create a safe platform to share information and ideas to bring the subject matter technology to an advanced level, MTAs allow the sharing of research materials, tools, or data to nourish the current technologies. CRADAs allow a more robust exchange of materials, information, and financial sources to generate better and more ideal solutions for unsolved problems in every dimension of the technology field. A Licensing Agreement allows for the establishment of a commercial collaboration for the development and commercialization of an invention.
As mentioned in Section 3 related to the history of AI, we are in the third wave and the third summer of AI.2-4 To better understand the current role of AI-based technologies in contract management, we searched companies involved in this field in the Pitchbook database. We plotted the graph of the number of companies over the years (Figure 4). According to the Pitchbook data, 81 companies work in some way in AI technology and contract management. We examined all 81 companies for their AI tools or services that are in use or can be used in the future for technology transfer agreements and other procedures. We found 55 companies out of 81 in the AI-based contract management field that can be used or be helpful for the technology transfer process.
The first company in an AI-based contract management field was founded in 1990 and developed AIbased software and ML algorithms to access critical insights from documents to help enterprises review and validate critical information in existing contracts and legal documents, enabling clients to apply context to content, replace ambiguity with clarity, and provide understanding even in the absence of structure in real time. AI’s involvement in contract management started during the third summer of AI, and the number of AIbased contract management companies peaked in 2017 and 2018. After 2018, there has been a decrease in the foundation of AI-based contract management companies (Figure 4.)
When we examined investment trends in the AIbased contract management field, we saw a minimal peak with a maximum investment of $23.48 million in 2014. In the same year, there was a drop in the foundation of AI-based companies in the contract management field. In 2017, while there was a slight increase in investment, there was the most significant jump in the number of new companies. On the other hand, while the number of newly founded companies is decreasing, the level of investment made in 2022 was $539.31 million. The trends in investment and in the number of newly established companies are different in the AI-based contract management field. One of the reasons might be that investment for expanding the current company is more crucial than establishing a new company for this AI field.
Therefore, we might say that AI-based contracts management technologies are in the AI winter, based on the Pitchbook data (Figure 4, Figure 5).
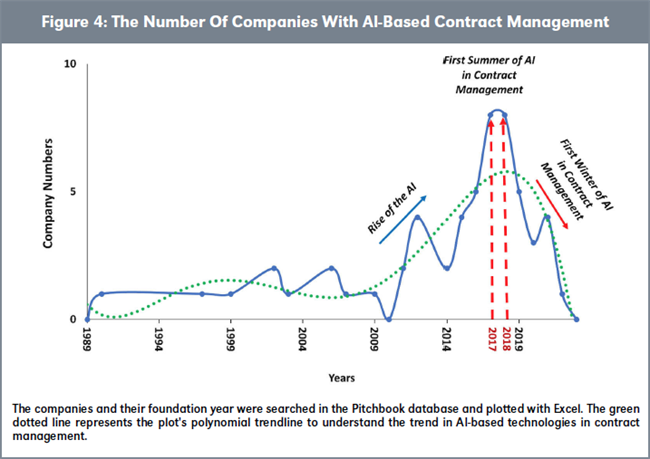
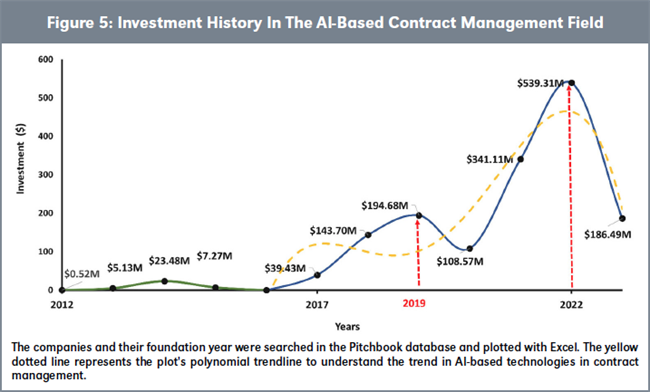
Technology transfer agreements create an excellent platform for collaboration to accelerate an invention development; on the other hand, these agreements are necessary for creating boundaries of cooperation for each side, including the intellectual property interests of collaborating parties underlying the legal protection plan of an invention and/or new scientific and technical information from public disclosure. A traditional simple agreement execution process consists of these listed steps as summarized in Figure 6.
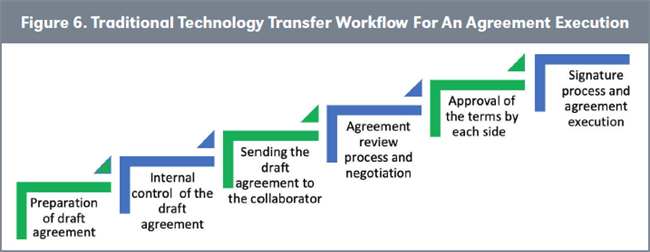
One of the significant applications of AI in technology transfer is contract management. After Open AI launched ChatGPT for public use in November 2022, many law firms in the U.S., U.K., and Canada became aware of AI’s capabilities in the legal field.5 There are currently law firms that use AI for managing contracts. All agreement execution steps listed in Figure 6 can be managed by available AI tools in the following process of contract management:6
- Drafting Contracts: AI can draft contracts based on standard terms and conditions. If a company needs to execute many standard contracts, AI will have great potential to save time and money.
- Negotiating and Reviewing Contracts: AI can compare specific clauses, identify potential disagreements and risks in the revised agreement, create automated redlines, and recommend suggestions. Currently, commercially available contract drafting, reviewing, and tracking software can generate automated redlined contracts faster than humans. AI can compare specific clauses, identify potential disagreements and risks in the revised agreement, create automated redlines, and recommend suggestions.
- Managing Contracts: AI can monitor the executed agreements, track the expiration term, and specific milestones, and remind regarding renewals. AI-mediated agreement tracking software is also commercially available.13-15
An ML system and hundreds of thousands of contracts are two key elements to train AI systems for accuracy in contract management.4, 16 A well-trained AI system can draft, review, and compare agreements, identify key clauses and risks, generate proposals and counteroffers, and create automated redlining.17 AI tools can also provide insights and recommendations based on data and best practices. AI tools can improve efficiency, accuracy, and consistency in agreement negotiation and save time and money. According to the currently discovered capabilities of Al, if the technology transfer experts train Al with various agreement types, AI can take over agreement execution steps, as listed in Figure 6.
Lawyers use AI-mediated contract software for clause identification, key-data-point identification, and automated redlining. While 23 percent of the midsize law firms with employee numbers between 11 and 179 currently use AI tools, only 10 percent of smaller law firms use them for their practices,7 since it is expensive to implement the AI system.
NYU Langone Health and Texas Tech University are two examples of AI-using transfer offices.
Currently, closed AI systems are rare among tech transfer offices due to high costs. New York University established a closed system to provide confidentiality for their AI system and trained AI to review up to 30 agreements per hour. The return of the AI system: based on their current experience and cost-benefit evaluation of the AI system, NYU considers that most tech transfer offices might be using AI systems within 18 months.
Texas Tech University uses an AI-based system for internal operations and proposal development. Texas Tech University considers that there are unforeseen sides to AI-based systems and recommends being cautious about inputting proprietary ideas or novel concepts into generative AI platforms, which could affect the patentability of an invention.
Even though many technology transfer offices consider the use of generative AI in their tech transfer work, they have concerns about open AI systems’ risk for confidentiality and the possibility of AI’s biased or even nonsensical “hallucinations.”18
5.3. Intellectual Property (IP) Protection: It has recently been shown that ChatGPT can write a provisional patent in less than one minute with a single-sentence invention disclosure and file the provisional patent application to the USPTO within hours. However, some points must be improved or addressed before including AI in patent writing and filing processes. First, AI could not be listed as an inventor on a patent application because the Patent Act (Title 35 of the U.S. Code) requires that inventors be natural persons. Therefore, ChatGPT cannot “invent” and does not “own” anything. Secondly, there is a high risk that ChatGPT might incorporate third-party copyrighted material or other third-party intellectual property.19 AI could easily help patent agents and attorneys with simple routine elements of the patent writing and filing process, but both a patent agent and an attorney still need to have the ultimate responsibility to review the whole process.
6. Advantages and Disadvantages of AI in Agreement Management
Although AI brings many advantages to the technology transfer field, AI still has aspects that need to be improved. In this study section, we discuss the advantages and disadvantages of AI in the technology transfer field.
6.1. Advantages of AI-based Technology Use in Technology Transfer
- Time Saving: AI-based tools can complete the following technology transfer tasks in a short period:
- Reviewing and analyzing large volumes of contracts.
- Drafting a regular contract or a provisional patent application in a short time.
- Filing a provisional patent application within hours.
- Completing marketing landscape analysis through extensive data file search within a short time.
- Error Reduction: AI-based tools can process an excessive amount of data with minimal errors and can eliminate human-caused errors.
6.2. Disadvantages of AI-based Technology Use in Technology Transfer
- Confidentiality Risk: One of the biggest concerns for AI-based tools is confidentiality since the firewall behind ChatGPT talks to OpenAI and eventually the whole world. Therefore, an AIbased system has difficulty in its adaptation due to its risky profile for data privacy, confidentiality, and security.11, 13, 14 It is suggested that one of the direct solutions for confidentiality might be to create a private ChatGPT behind a firewall that will not talk to either OpenAI or the world. This private ChatGPT might allow institutions to develop AI-based tools without confidentiality concerns.
- Accuracy: AI-based systems can create reliable outcomes if they are trained with good quality data. However, one of the biggest concerns of AI systems is the “AI Hallucination State.” If the AI system does not understand the questions, it can misinterpret and generate unrelated answers. This potential AI Hallucination State therefore requires extensive data training to be mitigated.8
However, one of the university technology transfer offices in the United States considers using the Large Language Model (LLM) as a solution for the hallucination problem of AI. LLM is an AI algorithm that uses deep learning to summarize, generate, and predict new content from enormous data sets.11 Using an LLM system with a smaller dataset and fewer options for the AI system to choose from can improve the hallucination problem and the accuracy of AI-based models. LLM systems have excellent adaptability, flexibility, and accuracy. They are also easy to train.20 If there is smaller data set usage, then this also might reduce data storage costs.
Today, AI systems do not have accurate functions in legal applications since there is no extensive and high-quality data to train AI-based legal tools.9, 10, 15 Therefore, the optimization process for AI-based systems used in legal applications is still at the very early stage.
- Bias: Bias is one of the biggest concerns regarding the AI-based system’s decision-making. It is interesting to imagine an algorithm has bias during its decision-making. However, there are many examples of bias in the AI systems.8 AI models’ application in legal,21 regulatory,22 and ethical oversight requires organizational accountability, decision rights, risks, policies, and investment decisions. For this purpose, the U.S. government proposed legislation, the Algorithmic Accountability Act of 2019. This bill requires companies to assess bias, effectiveness, and other factors to make critical decisions when using an automated decision system. Therefore, there must always be validation by human experts to move forward with AI-based decisions, and there should be improvements in AI algorithms to prevent biased decisions.
- Cost and Productivity: AI-based systems require both large and excellent quality data to perform accurate work. To be able to work with a large data set, there is a need for costly storage places.15 The performance of AI-based patent searching tools has not been found to be reliable due to the AI hallucination problem. Therefore, the subject expert must validate every AI work outcome, which also takes time and affects productivity.
- Need For Regulation and Policies In AI Systems: There is limited regulation, guidance, or policies for embedded personal data in AI-based systems.8 This might create a very big problem and trust concern for confidential agreements by using AI-based technology transfer tools.23 However, the U.S. government has taken several steps to prevent AI-based discrimination bias and promote trustworthy Al.
On December 8, 2020, Executive Order (EO) 13960, Promoting the Use of Trustworthy Artificial Intelligence in the Federal Government, was issued. The EO aims to prevent bias in the design and use of new technologies, including AI, and to protect the public from algorithmic discrimination and also aims to promote the use of trustworthy AI in the federal government.
In October 2022, the White House released the Blueprint for an AI Bill of Rights to help guide the design, development, and deployment of AI and other automated systems so that they protect the rights of the American public. The Blueprint consists of five principles, including (1) Safe and Effective System, (2) Algorithmic Discrimination Protections, (3) Data Privacy, (4) Notice and Explanation, and (5) Human Alternatives, Consideration, and Fallback.
More action still needs to be taken to make private industries follow government regulations and policies. There is also an urgent need for more global regulation and policies regarding the safe and ethical use of AI.
7. Discussion
AI has been reshaping every aspect of our daily lives, and it is currently either in use or under development to be used in most jobs all around the world. This study aims to uncover AI’s current and future role and usage in technology transfer.
Pitchbook’s data regarding the number of companies and investments in the AI-based contract management field shows that AI use in technology transfer is still premature. There are currently 38,969 companies in the AI field, and only 57 are in the contract management field, equaling 0.14 percent of the total AI company population. A closer look at the investment trend and newly established companies in the AI-based contract management field reveals a slowing down in new company establishment and investment. We might say that investment in AI-based contract management is still not currently a primary interest.
There is an emerging trial usage of AI in technology transfer offices. AI can draft and revise agreements, draft patents, search prior art, file a patent, and even involve targeted marketing of an invention that requires the most human interaction. However, all these processes need to be validated by a technology transfer specialist at the end.
Four critical elements need to be addressed in AI usage in technology transfer:
- Good quality data to train AI.
- Affordable housing for data storage.
- Well-established international and national policy to use AI in technology transfer.
- Preventative action to provide safety for confidential information of agreements.
If these four elements are provided, it is easy to predict that every technology transfer office will be using AI in any part of their daily jobs in the future. However, there will always be requirements for validating AI-based work by the technology transfer expert, patent agents, or lawyers.
8. Conclusion
In this study, we attempted to uncover AI’s current and future use in the technology transfer field. The current applications and trends of AI in contract management tell us that AI-based contract management tools still need more attention and improvement. Based on the current capabilities of AI, it will neither be able to replace lawyers nor technology transfer professionals as it functions today. However, AI with human cooperation and improved algorithms might be expected to significantly increase contract management efficiency in the technology transfer field. As technology transfer professionals, we will all need to carefully follow this rapidly evolving field! ■
References
- Flasiński, M., Introduction to Artificial Intelligence. 2016.
- Buchanan, B.G., “A (Very) Brief History Of Artificial Intelligence,” AI Magazine, 2005. 26(4): p. 53-53.
- Roser, M. The brief history of artificial intelligence: The world has changed fast—what might be next? https://ourworldindata.org/brief-history-of-ai, 2022.
- Sumit Das, A.D., Akash Pal, Nabamita Roy, “Applications of Artificial Intelligence in Machine Learning: Review and Prospect,” International Journal of Computer Applications, 2015. 115 (9): p. 31-41.
- Surden, H., “The ethics of artificial intelligence in law: Basic questions.” Forthcoming chapter in Oxford Handbook of Ethics of AI, 2020: p. 19-29.
- Marr, B., Understanding the 4 Types of Artificial intelligence. https://bernardmarr.com/understanding- the-4-types-of-artificial-intelligence/, 2021.
- Setchi, R., et al., “Artificial intelligence for patent prior art searching,” World Patent Information, 2021. 64.
- Privacy Preserving AI. in United States and European Union Artificial Intelligence Engagement Series- National Cancer Institute (NCI). 2023. Virtual Conference.
- Huang, M.-H. and R.T. Rust, “A Strategic Framework For Artificial Intelligence In Marketing,” Journal of the Academy of Marketing Science, 2021. 49: p. 30-50.
- Abid Haleem, M.J., Mohd Asim Qadri, Ravi Pratap Singh, Rajiv Suman, “Artificial intelligence (AI) applications for marketing: A literature-based study,” International Journal of Intelligent Networks 2022. 3: p. 119-132.
- AUTM Artificial Intelligience Discussion Forum. 2023.
- Uygur, B., S. Ferguson, and M. Pollack, “Hiding In Plain Sight: Surprising Pharma And Biotech Connections To NIH’s National Cancer Institute,” Journal of Commercial Biotechnology, 2022. 27(2): p. 5.
- Macey-Dare, R., How ChatGPT and Generative AI Systems will Revolutionize Legal Services and the Legal Profession. Available at SSRN, 2023.
- Institute, T.R. ChatGPT and Generative AI within Law Firms. 2023.
- https://ironcladapp.com/journal/contract- management/generative-ai/. Generative AI and How it Improves Contract Management. 2023.
- Khanzode, K.C.A. and R.D. Sarode, “Advantages and disadvantages of artificial intelligence and machine learning: A literature review,” International Journal of Library & Information Science (IJLIS), 2020. 9(1): p. 3.
- How GCs Can Leverage AI to Negotiate Contracts. https://www.law.com/corpcounsel/2023/04/26/ how-gcs-can-leverage-ai-to-negotiate-contracts/.
- AUTM. Navigating AI Now Could Pay Big Dividends Later for Your TTO. 2023.
- Dorius, K., AI Wrote My Patent Application in 30 Seconds and It Is Now Pending with the USPTO and Will Soon Be Licensed. 2023, Linkedin: https:// www.linkedin.com/pulse/ai-wrote-my-patent-application- 30-seconds-now-pending-kirk-dorius/?trackingId= sr34hAfLT7CHCegtsVFQFw%3D%3D.
- Kerner, S.M., Large Language Models (LLMs) 2023, https://www.techtarget.com/whatis/definition/ large-language-model-LLMlarge.
- Coglianese C, L.D., “Transparency and Algorithmic Governance,” Administrative Law Review 2019. 71(1): p. 18-38.
- U.S. Food & Drug Administration. Proposed Regulatory Framework for Modifications to Artificial Intelligence/Machine Learning (AI/ML)-Based Software as a Medical Device (SaMD): Discussion Paper and Request for Feedback. https://www.fda.gov/media/ 122535/download.
- Nguyen, Y.S., “Artificial Intelligence Contract: How Algorithms and Machines have Disrupted the Way Law is Practiced,” PM World Journal, 2019. 8(9).